If you enjoy this post please share to widen the discussion and consider subscribing for weekly articles to your inbox!
2019 was filled with build up and promise for the potential of AI, it looks as though the narrative in 2020 is taking a different tact. There’s been an increasing number of articles already in 2020 questioning if another AI winter is coming. An AI winter is where there’s a period of reduced funding, research and development. Whether this is the media cycle looking to create a fresh angle or it's a reality time will tell. What we do know is 2019 was the year for record investment into AI, also in the first 2 months of 2020 we've had NHS AI Award confirmed guaranteeing funding over 3 years, the EU AI white paper and ongoing investment from big tech all this momentum is not going to just drop. Its unlikely that we have an AI winter on the horizon but instead a change in the narrative with more focus on the "failings" of AI. In reality AI cannot live up to the hype (we don’t have AI doctors) and the AI we have is narrow - very good at very specific things in specific situations. That was to be expected but got lost in the hype. Instead the reality of AI limitations are now starting to be discussed. The shift away from hype is a turning point that has the potential to enable us to progress on the hard parts of AI which can ensure the foundations are developed to create the best AI and address a evangilise v demonise toxic AI culture that's being created.
Human-AI co-working
AI co-working is an area that is fundamental to the successful implementation of AI. How do we create AI that works in a way that supports healthcare professionals to improve care and support clinical judgement making? We need tools to identify the bias of both human and AI and mitigate this. What is the AI user interface? Information needs to be provided in an actionable way but also needs to be trusted by the clinician who wants to avoid unnecessary risk to patients. AI are complex software algorithms this complexity needs to be abstracted to provide tools that are reliable and transparent - communicating in a trustable and evidence based approach is paramount. How does AI modify subconcious clinician behaviour? With AI tools providing information potentially conveyed with nudge based wording in combination with black box complex systems can alter clinical behaviour. This will require different training to support clinicians to objectively utilise such tools.
Correlation v causation: AI is very powerful software but it’s also narrow and lacks context. This means it can make correlation between data but can’t reason if there is a causal link. There’s a risk of AI providing insights based on correlation not causation resulting in a misuse of resources or potentially even harm. The work on building causation into AI algorithms is still early stage and limitations need to be accounted for. AI may need to be able to convey assumptions on context and clinicians may need to be able to navigate this additional degree of complexity, which then begs the question is the AI adding more complexity than it solves?
Crystallisation: AI has the potential to make guidelines which currently support clinical decision making and biases that exacerbate inequality of care into the rule of care provision. This means a higher consistency but removes the clinical autonomy and clinical judgement. It may also may mean crystallisation of bias, processes and disease treatment approaches. How do we change the AI if pathways or processes change without the data to train the software?
Learnings from self driving cars: Approximately 10 years ago we were promised autonomous vehicles. Significant progress has been made but we’re still a far stretch from a self driving car. Instead we have many assistive features and a much better idea of what is defined as an autonomous vehicle and a true understanding of how complex it is. Autonomous vehicles were seen as a deterministic problem that could be easily solved but in reality it’s much more complex. As a result there have been deaths and injuries. What we now have is a much better idea of what autonomous means and what the stages are to get there (see figure below - we're only at stage 2/3). We’re not even close to this clarity of AI scope in healthcare - healthcare is magnitudes more complex than driving with psychological, biological, environmental and many other factors to consider both in the immediency and over long term.
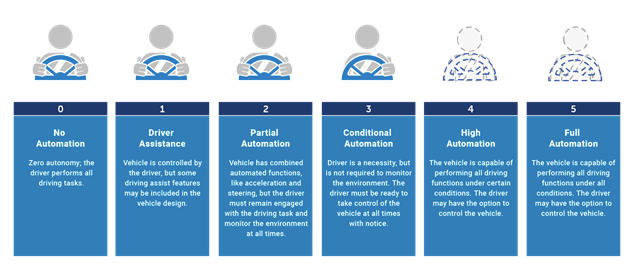
Autonomous vehicles have demonstrated that in health we need to map and define AI for each application perhaps even to the point of individual tasks. Where can we have full AI control, where do we need to enable seemless person-AI transition and where should a person only be involved? How should individual choice be incorporated? are all unanswered questions.
Turning the AI corner
The media narrative means a fresh angle on AI is being sought. There's sufficient investment and momentum behind AI that it's unlikely to turn into an AI winter but we may be entering a period of disappoingment or a good opportunity to reset expecations. This means we can progress with getting the frameworks and basics right to build AI functionality considering user interface, layers of AI within each bundle of tasks and accounting for narrowness and limited context in software. This also presents the opportunity to start tackling the cultural impact of the AI hype.
Enjoyed this post? Do share with others! You can subscribe to receive posts directly via email.
Get in touch via Twitter or LinkedIn.
Quick links to recent interesting articles:
- In 2019 poor healthcare IT security has cost $4B. Increasing prevalence and cost of poor cyber security in healthcare
- Early signs of cancer can appear years or even decades before diagnosis
You may also like:
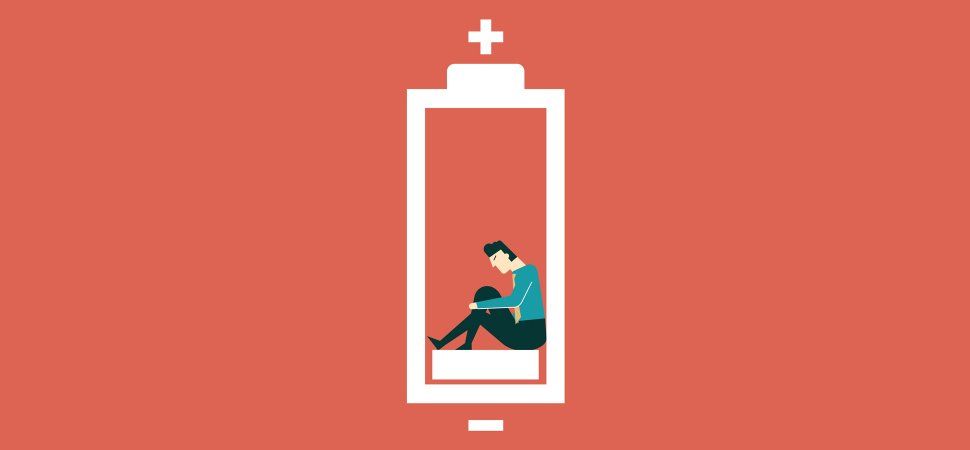
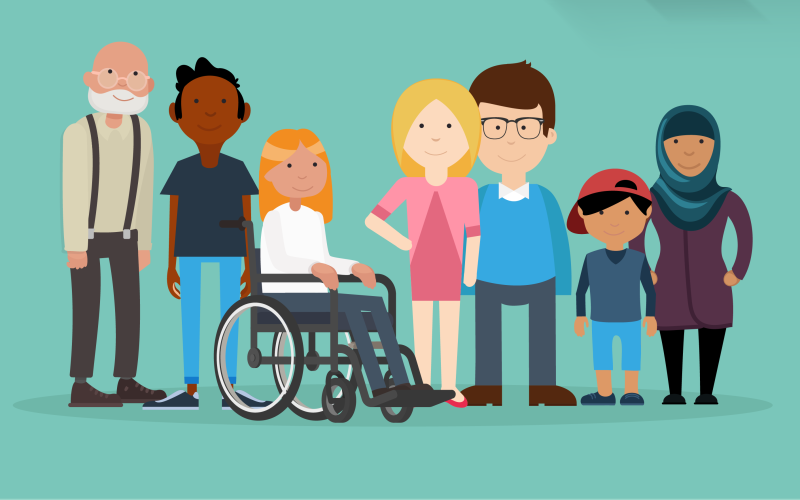
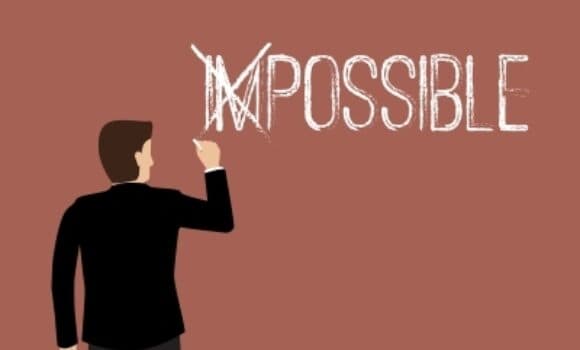